A survey of validity and utility of electronic patient records in a general practice*
A Hassey, D Gerrett, A Wilson
HK Pract 2001;23:540-547
Summary
Objective: To develop methods of measuring
the validity and utility of electronic patient records in general practice.
Design: A survey of the main functional areas
of a practice and use of independent criteria to measure the validity of the practice
database.
Setting: A fully computerised general practice
in Skipton, north Yorkshire.
Subjects: The records of all registered practice
patients.
Main outcome measures: Validity of the main functional areas
of the practice clinical system. Measures of the completeness, accuracy, validity,
and utility of the morbidity data for 15 clinical diagnoses using recognised diagnostic
standards to confirm diagnoses and identify further cases. Development of a method
and statistical toolkit to validate clinical databases in general practice.
Results: The practice electronic patient
records were valid, complete, and accurate for prescribed items (99.7%), consultations
(98.1%), laboratory tests (100%), hospital episodes (100%), and childhood immunisations
(97%). The morbidity data for 15 clinical diagnoses were complete (mean sensitivity=87%)
and accurate (mean positive predictive value=96%). The presence of the Read codes
for the 15 diagnoses was strongly indicative of the true presence of those conditions
(mean likelihood ratio=3917). New interpretations of descriptive statistics are
described that can be used to estimate both the number of true cases that are unrecorded
and quantify the benefits of validating a clinical database for coded entries.
Conclusion: This study has developed a method and
toolkit for measuring the validity and utility of general practice electronic patient
records.
Introduction
The NHS and its workforce are being made accountable for the services they provide
through the emerging mechanisms of clinical governance.1,2 These mechanisms
will depend crucially on the availability of high quality health information in
clinical practice,3,4 and such data will need to be accessible through
electronic patient record systems.5
These factors led us to consider the validity and utility of the electronic patient
record system in a general practice and how these might be measured. The principal
aim of this study was to measure whether the practice's electronic patient records
were a true record of the health events associated with the patients of the practice.
The prime function of the medical record is to support patient care.5
The general practice record is based on an individual and is a contemporaneous list
of entries about that person's health. Record entries in computerised general practice
systems generally consist of a mixture of text and Read codes. Together these form
the narrative structure and content of the electronic patient record.
Measures of validity tell us whether an item measures what it is supposed to that
is, whether a measurement is true.6 An example would be to test whether
the presence of the Read code for diabetes in the database truly means that the
patient has diabetes.
Reliability refers primarily to the consistency or reproducibility of the data or
test. The degree of reliability of the measures applied to the data will set limits
on the degree of validity that is possible. Reliability is usually measured by the
degrees of correlation between measures of data. Reliability and validity must both
be in place to enable useful comparisons of sets of data to take place.
For the purpose of this study we extended Neal etal's definition of record validity:
"Medical records, whether paper or electronic, record health events. Records are
valid when all those events that constitute a medical record are correctly recorded
and all the entries in the record truly signify an event".7
Attempts to validate electronic patient record systems have usually involved validating
the database against either a paper record or patient survey.8-13 Sensitivity
and positive predictive value have been used as measures of completeness and accuracy
of recording respectively.8-10 In Britain the primary care information
services (PRIMIS) project was designed to help primary care organisations improve
patient care through the effective use of their clinical computer systems.14
PRIMIS uses a methodology based on standard MIQUEST queries15 to interrogate
practice clinical databases. These queries include validation checks, but they are
primarily useful as a tool for assuring the reliability of health data and facilitating
the analysis of aggregated anonymised datasets. We could find no other published
accounts of attempts to validate electronic patient records based solely on the
contents of the clinical database.
Subjects and methods
The study practice, Fisher Medical Centre, is based in Skipton, serving 13500 patients
in the Yorkshire Dales. The practice has used the EMIS clinical system since 1990
and has been "paperless" since 1994. Patient data are entered by general practitioners,
practice nurses, administrative staff, and attached community nurses. All patient
events and contacts with the practice should be recorded by direct entry, electronic
scanning of letters, or clinical messaging from local NHS providers (such as laboratory
reports). Much time and effort have been spent fostering an "information culture"
in the practice over the past six years (see Box 1).16
The practice clinical database should be valid, complete, and accurate since 1994.
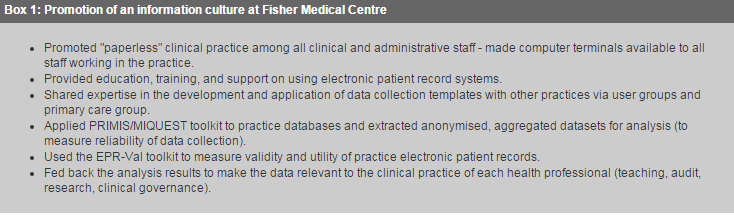
We performed our study (which was approved by the local ethics committee) in two
stages. The first stage was to build confidence in the validity of the clinical
database across the main functional areas of the electronic patient records (Table
1). We sampled practice activity retrospectively, so staff were not
alerted to the study beforehand and had no chance to change their recording behaviour.
We sampled the details of all patient events and contacts with the practice over
a typically busy week. We selected the study week at random but excluded weeks containing
a bank holiday. We validated the practice database for registrations and items of
service against the local health authority's database over three months. We also
measured the completeness and accuracy of practice prescribing with a one month
retrospective survey of the town's busiest local pharmacy. All prescriptions are
issued via the practice computer except those issued when working for the general
practice cooperative or on house calls. We surveyed the pharmacy to measure the
number of practice prescriptions dispensed by them and checked all handwritten prescriptions
against the practice electronic patient records. Finally, we compared the separate
record systems used by the practice's health visitors for preschool children with
the practice system to identify and check the vaccination status of all 3 years
old children in 1999.
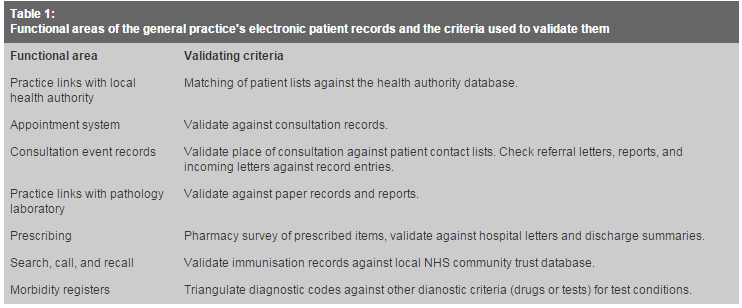
In the second stage of our study we measured the validity of the clinical entries
in the practice's electronic patient records. The principal innovation in our study
was to consider the Read codes in the records as tests for the true presence or
absence of the associated conditions in the database. The method was based on using
Read coded entries over the previous five years (or previous year for asthma and
ischaemic heart disease) to validate available criteria in the electronic records
for 15 clinical diagnoses (for details, see extra information on the BMJ's website).
These criteria would act as the standard for each diagnosis. We developed search
strategies for these conditions and tested them against a standard PRIMIS toolkit.14
We chose the 15 diagnoses on the basis that they represented important causes of
morbidity across the spectrum of chapter headings in ICD-9 (international classification
of diseases, ninth revision), that they could be validated against other criteria
in the patient record (drug treatment or diagnostic test), and that comparative
data were available from other published studies.
Statistical analysis
We used standard statistical tests to compare the practice database with the validating
criteria and other data sources such as the Morbidity Statistics from General Practice:
Fourth National Study.17 We measured the completeness and accuracy of
the electronic patient records in terms of sensitivity and positive predictive value
respectively. These statistics can be calculated from a simple 2?2 table and can
be applied to any "test" as a measure of its usefulness.18,19 The figure
in Box 2 shows a worked example for diabetes.
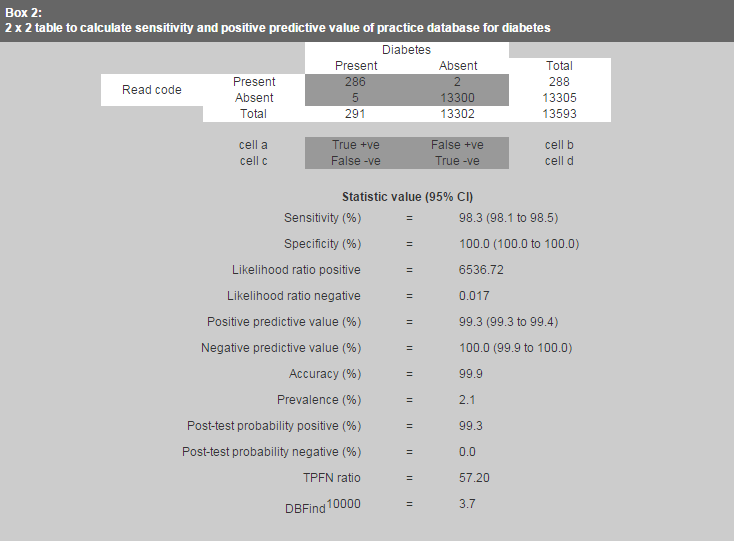
The power of a test can be understood in terms of its ability to change the prior
(pre-test) probability that a patient does or does not have the test condition.20,21
The positive predictive value gives the power of a test to change the probability
that the patient has the test condition. The likelihood ratio for a positive test
is the odds that the test will be positive in a patient with the condition compared
with a patient without the condition. The pre-test probability for any test condition
is the prevalence of that condition in the community. Likelihood ratios are an accepted
method of "testing tests".22,23 In this study they represent a quantifiable
measure of the validity of the Read coded entry to predict the true presence or
absence of the associated condition.
We checked valid data against recognised diagnostic standards for each condition
to confirm existing diagnoses and identify potential further cases. The difference
between the number of conditions existing in the database and the total number identified
was made comparable through development of two new descriptive statistics, the TPFN
ratio and the DBFind10000 (See Box 3 for details). We developed a toolkit
in Excel (EPR-Val) to calculate the full range of statistics (including the TPFN
ratio and DBFind10000) from the test data.
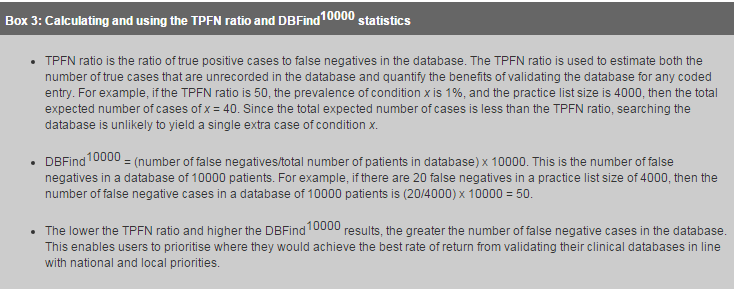
Results
Validity of the main functional areas of the electronic patient records
The practice list and all claims payments were fully reconciled with the health
authority over three months in 1999. These payments are an important check on validity
because they include procedures that can be carried out only on patients of the
appropriate age and sex. During the study week, we checked all appointments and
visits with practice clinicians against the database to confirm that every appointment
had a consultation entry: 98.1% of 1029 consultations were recorded in the clinical
records. Of the 20 not recorded, 12 were "Did not attend", and eight consultations
were missed. During the study week, the practice received 202 hospital letters,
358 pathology reports, and 12 contact sheets from the general practice cooperative
and made 44 referrals. There were several minor transcription errors, but clinical
details were correctly recorded in every case.
When we surveyed Skipton's busiest pharmacy we found a total of 639 practice prescriptions
were dispensed by them over one month, 629 computer generated and 10 handwritten
prescriptions. Of the handwritten ones, eight were properly recorded in the computer
clinical record and two were missed. Overall 99.7% of prescriptions tracked were
recorded by the electronic patient record during that month.
When we checked the vaccination status of all 3 year old children in 1999 we produced
a list of 144 children. The records matched with the separate record systems used
by the practice's health visitors in 140 (97%) cases, the remaining four children
had just registered with the practice and were unknown to the health visitors.
Validity of clinical entries in electronic patient records
We ran a series of standard MIQUEST validation queries, which included checks on
codes that are sex specific. Two men were recorded as having had cervical smears,
but there were no other unreconciled procedural coding errors. This gave us the
confidence in our coding to populate the 2?2 contingency tables (details available
on the BMJ's website) and calculate the statistics for each of the 15 diagnoses
(Table 2). These results show that the practice database was valid,
complete, and accurate. The results for obesity are an exception and reflect a coding
practice of recording body mass index rather than the diagnosis of obesity. The
likelihood ratios indicated that the presence of the Read codes for the 15 conditions
indicated a true diagnosis in 96% of cases. The absence of the Read code indicated
the true absence of those conditions in 99.5% of cases (Table 2).
Table 2: Summary
statistics produced by EPR-Val toolkit for 15 test diagnoses
|
Test condition
|
Prevalence (%)*
|
Sensitivity (%)
|
PPV and PTP +ve (%)
|
LR +ve
|
LR -ve
|
PTP -ve (%)
|
TPFN ratio
|
DBFind10000
|
|
|
|
|
|
|
|
|
|
Breast cancer
|
0.4
|
94.7
|
100
|
|
0.053
|
0.0
|
18.0
|
2.2
|
Prostate cancer
|
0.2
|
100
|
96.0
|
13577
|
0.00
|
0.0
|
|
0
|
Diabetes
|
2.1
|
98.3
|
99.3
|
6536
|
0.017
|
0.0
|
57.2
|
3.7
|
Obesity
|
6.0
|
2.1
|
89.5
|
134
|
0.979
|
5.8
|
0.02
|
583.7
|
Hypothyroidism
|
1.7
|
82.1
|
97.5
|
2196
|
0.179
|
0.3
|
4.6
|
30.9
|
Hyperthyroidism
|
0.4
|
97.9
|
95.9
|
6632
|
0.021
|
0.0
|
47.0
|
0.7
|
Gout
|
1.6
|
93.7
|
97.6
|
2507
|
0.063
|
0.1
|
14.8
|
10.3
|
Iron deficiency anaemia
|
1.4
|
77.6
|
96.8
|
2080
|
0.224
|
0.0
|
3.5
|
31.6
|
Glaucoma
|
0.8
|
96.4
|
95.5
|
2601
|
0.036
|
0.0
|
26.8
|
2.9
|
Epilepsy
|
0.6
|
94.0
|
95.2
|
3179
|
0.060
|
0.0
|
15.8
|
3.7
|
Parkinson's disease
|
0.2
|
86.7
|
96.3
|
11760
|
0.133
|
0.0
|
6.5
|
2.9
|
Ischaemic heart disease
|
5.2
|
95.5
|
97.1
|
615
|
0.045
|
0.2
|
21.3
|
23.5
|
Hypertension
|
6.6
|
97.8
|
98.9
|
1242
|
0.022
|
0.2
|
43.6
|
14.7
|
Asthma
|
5.3
|
87.3
|
86.3
|
112
|
0.128
|
0.7
|
6.8
|
67.7
|
Rheumatoid arthritis
|
0.7
|
98.9
|
92.2
|
1670
|
0.011
|
0.0
|
94.0
|
0.7
|
Average for all diagnoses
|
-
|
87
|
96
|
3917
|
0.1314
|
0.05
|
35.7
|
51.9
|
The TPFN ratios and DBFind10000 results for asthma, iron deficiency anaemia,
hypothyroidism, and ischaemic heart disease indicate high priority areas for the
practice to identify previously undiagnosed true cases of these conditions in the
database.
Discussion
The first stage of this study established a method for validating a general practice
electronic patient record system. The study period covered just one-geek, and other
random checks might be important for particularly busy times. However, we are confident
that our sample was representative of typical practice activity. The practice database
was generally valid for prescribed items, consultations, laboratory tests, hospital
episodes, and childhood immunisations. The results compare favourably with those
of other published studies.9,13,17
The second stage of the study measured the validity and utility of the clinical
database for 15 diagnoses. The morbidity data associated with these conditions were
highly valid and reliable. The prevalences of these diagnoses in this study were
generally higher than those reported in the Morbidity Statistics from General Practice:
Fourth National Study17 (see Table 3).
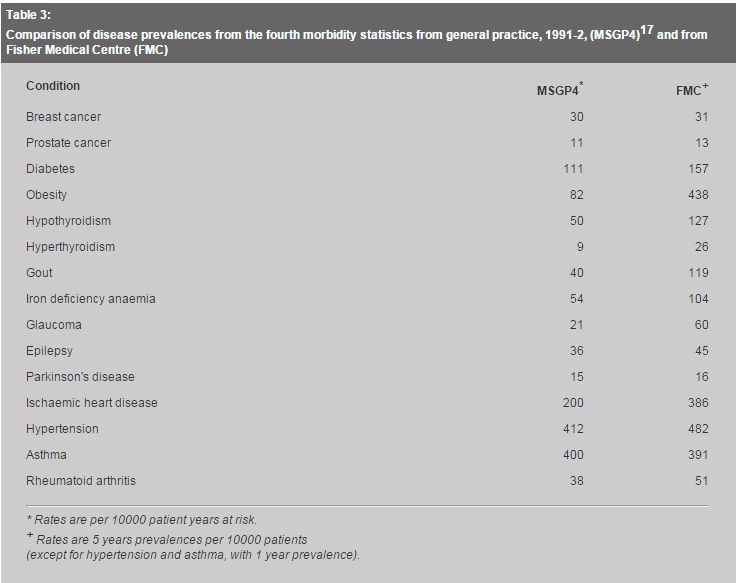
Our study assessed the power of a diagnostic code (Read code) to alter the probability
that a patient actually had a test diagnosis through the calculation of a range
of statistics. Sensitivity, positive predictive value, and likelihood ratio are
useful in combination to assess the overall validity of clinical diagnostic coding
in an electronic patient record because of their different strengths and weaknesses.18-20
We suggest that overall validity of electronic patient records should be assessed
with these measures in combination.
Health workers could use the method and toolkit described here to quantify the validity
of their electronic patient record systems. The derived statistics TPFN ratio and
DBFind10000 facilitate the estimation of the true prevalence of medical
conditions in the database, based on setting clinical criteria, and help quantify
the benefits of validating the database for each condition. Users could then prioritise
where they would achieve the best rate of return from developing and validating
their clinical systems in line with national and local priorities.
The statistical tests applied in this study are sensitive enough to enable health
professionals to measure the degree of confidence they can have in clinical coding
at the level of a single practice. A validation toolkit (EPR-Val) was developed
as part of the research project. This provides a full range of statistical tests
(including the TPFN ratio and DBFind10000), and we have made it freely
available on the BMJ's website.
In conclusion, we have developed a new approach to the validation of clinical databases
in general practice. We have validated a general practice electronic patient record
system and developed a standard method and toolkit for quantifying the validity
and utility of data in clinical databases. The results of this study are relevant
to all those involved in patient care and performance management in the "New NHS".
We thank Dr James Newell for providing independent statistical advice on the methods
developed and applied in this paper, Dr Richard Neal for his support through the
Yorkshire Research Network (YReN) and for his comments on the draft manuscript,
Dr Nick Booth for his advise, support, and encouragement; and Ms Sheila Teasdale
for her advice and encouragement.
Contributors: All three authors conceived and planned the study. AH and DG developed
the toolkit and performed the analysis. AH was the main author of the paper, with
revisions from AW and DG. DG will act as guarantor for the paper. In addition, Dr
David Pearson helped in the planning of this study and provided constructive criticism
and comments on the draft manuscript and Dr John Williams helped in checking and
correcting the EPR-Val toolkit.
Funding: The Fisher Medical Centre is a research practice funded by Northern and
Yorkshire Region of the NHS Executive.
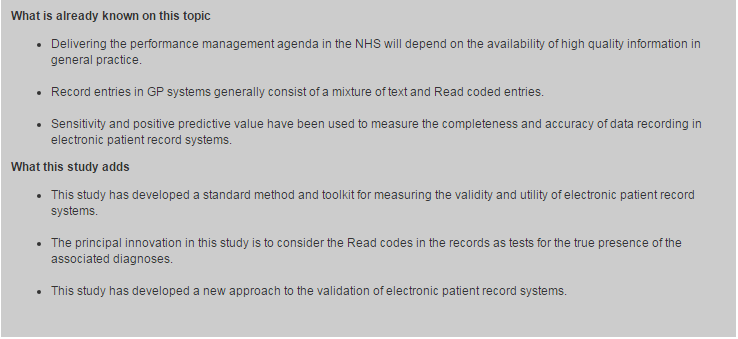
A Hassey,, General Practitioner, Fisher Medical Centre.
D Gerrett,, Senior Research Fellow,
School of Health and Community Studies, University of Derby.
A Wilson,, Senior Associate Lecturer,
Research School of Medicine, University
of Leeds.
Correspondence to: Dr A Hassey, , Fisher Medical Centre, Millfields,
Skipton BD23 IEU, United Kingdom.
References
- The New NHS. Modern, Dependable. London: Stationery Office, 1997.
- National Service Framework for Coronary Heart Disease. London: Department
of Health, 2000.
- The NHS Plan - a Plan for Investment, a Plan for Reform. London: Department
of Health, 2000 (www.nhs.uk/nationalplan/).
- Building the Information Care - Implementing the NHS Plan. London: Department
of Health, 2001
(www.doh.gov.uk/nhsexipu/strategy/overview/index.htm).
- NHS Executive. ScopeEPR: Royal College of General Practitioners Health Informatics
Task Force electronic patient record study. www.schin.ncl.ac.uk/rcgp/scopeEPR/report/index22.htm
(updated 13 Jul 2000).
- Kirk J, Miller ML. Reliability and Validity in Qualitative Research London:
Sage, 1986.
- Neal RD, Heywood PL, Morley S. Real world data retrieval and validation of consultation
data from four general practices. Fam Pract 1996;13:455-461.
- Whitelaw FG, Nevin SL, Milne RM, et al. Completeness and accuracy of morbidity
and repeat prescribing records held on general practice computers in Scotland. Br
J Gen Pract 1996;46:181-186.
- Whitelaw F, Nevin S, Taylor R, et al. Morbidity and prescribing patterns for the
middle-aged population of Scotland. Br J Gen Pract 1996;46:707-714.
- Mant J, Mant F, Winner S. How good is routine information? Validation of coding
for acute stroke in Oxford hospitals. Health Trends 1997-8;29(4):96-99.
- Pringle M, Ward P, Chilvers C. Assessment of the completeness and accuracy of computer
medical records in four practices committed to recording data on computer. Br J Gen
Pract 1995;45:537-541.
- Wilson AE, Pollock C, Weekes T, et al. Can general practice provide useful
information? - Evaluation of a primary health care information project in northern
England. J Epidemiol Community Health 1995;49:227-230.
- Pearson N, O'Brien J, Thomas H, et al. Collecting morbidity data in general
practice: the Somerset morbidity project. BMJ 1996;312:1517-1520.
- NHS Information Authority. PRIMIS (Primary Care Information Services). www.primis.nottingham.ac.uk/(updated
3 May 2001).
- Royal College of General Practitioners Health Informatics Task Force. MIQUEST and
health query language.
www.schin.ncl.ac.uk/rcgp/scopeEPR/nhs/Miquest/miquest.htm (accessed 4 May 2001).
- Teasdale S, Bainbridge M. An adult learning model for improving information management
in family practice. In: Cimino JJ, ed. Proceedings of the 1996 AMIA Annual Fall Symposium.
Philadelphia: Hanley and Belfus, 1996.
- Royal College of General Practitioners. Office of Population Censuses and Surveys.
Department of Health and Social Services. Morbidity Statistics from General Practice:
Fourth National Study, 1991-92. London: HMSO, 1995. (Series MB5 No. 3).
- Altman DG, Bland JM. Diagnostic tests 1: Sensitivity and specificity. BMJ
1994;308:1552.
- Altman DG, Bland JM. Diagnostic tests 2: Predictive values. BMJ 1994;309:102.
- Sackett DL, Richardson WS, Rosenbery W, et al. Evidence-based Medicine -
How to Practice and Teach EBM. London: Churchill Livingstone, 1997.
- Lilford RJ, Braunholtz D. The statistical basis of public policy: a paradigm shift
is overdue. BMJ 1996;313:603-607.
- McWhinney IR. A Textbook of Family Medicine. 2nd ed. Oxford: Oxford University
Press, 1997.
- Greenhalgh T. How to Read a Paper. London BMJ Books, 1997.
|